The Problem with Pre-Employment Assessments
Nov 20, 2020
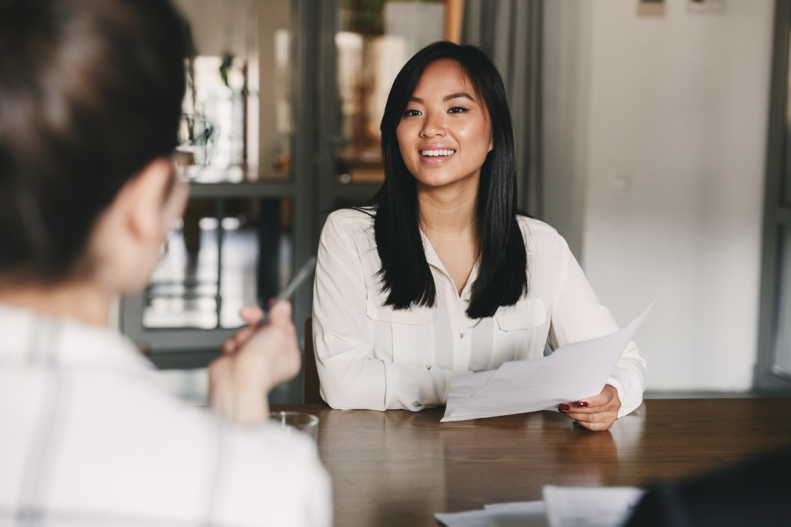
Pre-employment tests — screenings, assessments — have an esteemed history dating back to the evaluation of American soldiers in WWI.
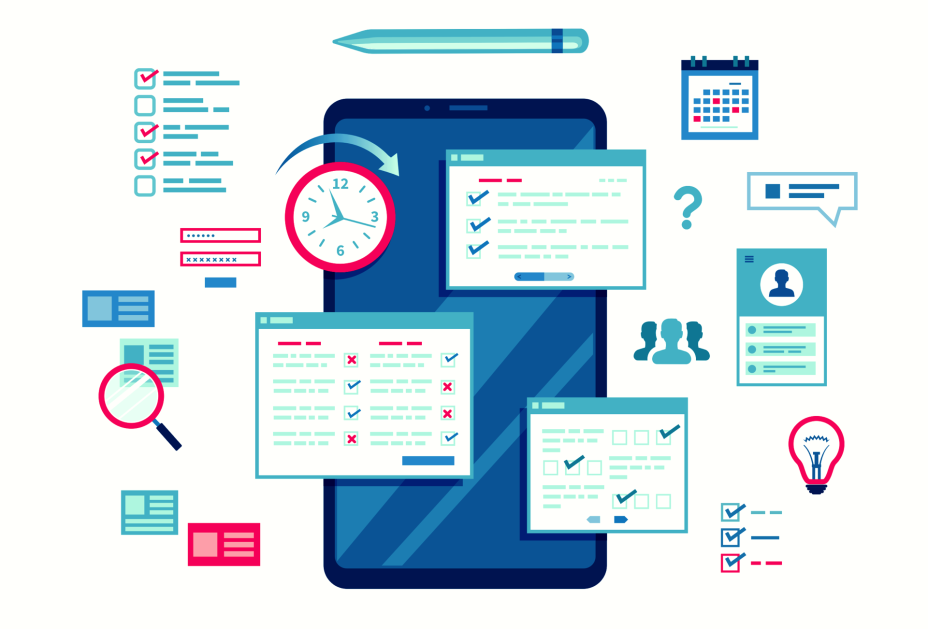
Today, many of the decades-old assessment companies have updated their descriptions and websites. They claim they leverage Data Analytics and Artificial Intelligence. They also claim to impact the outcomes deemed most important by their client organizations.
All of these claims must be carefully examined. They are not often supported by data.
We are 20 years into the 21st century. It’s time we evaluate the evaluation tools of the 1900’s. Today’s pre-employment screening tools should provide data-rich evidence of measurable impact. A seal of approval from I/O Psychology and ‘metrics of validity’ were once sufficient, but metrics of efficacy have evolved and our expectations from hiring processes should, too. If we cannot calculate ROI on a tool, then it may only be providing us with sentimental value.
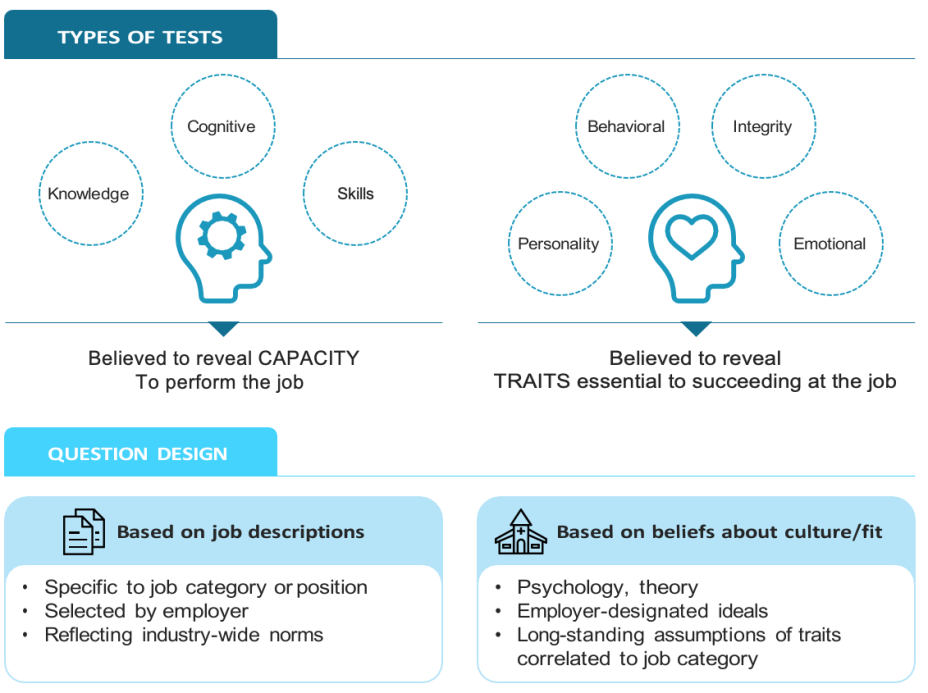
What do Assessments Reveal?
Assessments ask applicants questions and then compare their answers to ‘ideal’ (aka correct) answers.
Assessment questions are designed to reveal the capacity of an applicant to succeed at a job. However, two of the three EEOC-approved metrics of validity are not tied to actual job performance. Content Validity and Construct Validity provide circular metrics. They examine the extent to which a question measures what it claims to measure. Questions can attempt to measure a piece of knowledge, a skill, an attitude or trait that is presumed to impact job performance. But whether this presumption is accurate — whether the question actually relates to job performance — is not part of the validation process.
The third approved approach to validity is Criterion-related. This does attempt to correlate high scores on a test with success at a job. The approach is either:
Concurrent: examines scores of ‘top performing’ current employees, or
Predictive: examines scores of applicants who were hired and later deemed to be performing well on the job
Assessment companies do not track the performance of their clients’ new hires and, therefore, cannot claim true Predictive validity. It is more likely that assessment companies have, at some point in time, ‘validated’ their assessments by testing workers whom employers indicated were top performers. While this may provide a degree of reassurance, it is unclear what ‘top performance’ means. One cannot assume this designation aligns to job success at a different place of work, under different conditions, or at a different point in time.
The Predictive Problem
According to research synthesis in the Harvard Business Review, “a century’s worth of workplace productivity data” affirmed that Personality Pre-hire Tests are the least effective hiring practice in predicting job performance. On a scale from 0-1, with larger numbers indicating higher correlation between test score and job performance, Personality Tests scored 0.22, the second lowest of any hiring selection practice. The next least effective method was Emotional Intelligence Tests (0.24), followed by Integrity Tests (0.46).
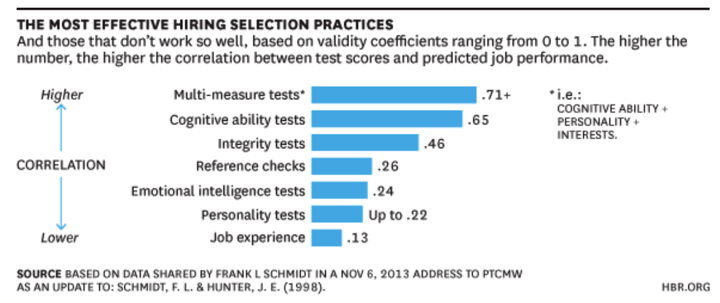
These tests are weak predictors for a number of reasons. People can answer these questions to reflect what they think are ideal traits, not necessarily their true traits. The presumption that a trait — in of itself — can predict job performance is a large leap. Tests fail to connect theory with the reality of a context influenced by people and personalities, workplace culture and constraints, unique challenges and unexpected changes.
Cognitive Tests were found to provide the highest predictive value (0.65) of any assessment. Unlike the other pre-hire tests, which had little difference among subgroups of job categories, Cognitive tests produced a wide range of predictive values depending on job complexity. The high predictive values only hold for highly complex jobs. For low to medium complexity jobs, predictive values hover between 0.24-0.51. Other considerations have been found to weaken the predictive value for Cognitive and other skills and knowledge tests:
People with the capacity to do a job well, may not choose to do the job well under certain circumstances
People with the capacity to do a job well in a theoretical reality, may not be able to do the job well under actual circumstances and employer-specific realities
People that do not currently possess the necessary cognitive skills may be able to easily acquire these skills and perform well at the job because they already possess the other necessary traits which are not as easily learned but are essential to the role
For all these reasons, a Cognitive test can regularly provide inappropriate guidance to hiring managers and talent acquisition professionals.
Is There a Benefit to Pre-Hire Assessments?
Assessment tools can provide visibility into an applicant’s skills, capabilities, or personality. Getting acquainted with these aspects of an applicant can help hiring managers interview applicants and develop a feeling of connection to new hires. It may allay hiring managers’ anxiety about welcoming a stranger to the team.
For highly complex roles that require the new hire to hit the ground running, a cognitive test that includes work task simulations could be the perfect tool to ensure that significant training and onboarding will not be needed. As Schmidt’s research (summarized in the chart above) indicated, cognitive tests had a high predictive value for highly complex jobs.
If one is seeking a reliable prediction related to future performance, however, a 20th century assessment is not the appropriate tool.
The 21st Century Solution to Problematic Assessments
Data analytics can do the heavy lifting of analyzing outcomes related to new hires that businesses wish to optimize – performance outcomes, retention, engagement, or any other measurable metric. A machine learning platform can work through continuous data flows from the employer and the local labor market, and then identify the job applicants who are most likely to produce similar outcomes in similar jobs/departments.
Once employers select outcomes to optimize, Arena’s machine learning platform can integrate into the hiring process and begin analyzing applicants, their own recent hires, and the data sources that Arena mines to build predictive algorithms. Recruiters and hiring managers need not change their existing workflows – they simply receive prediction scores alongside all other applicant information.

It is Arena’s outcome data that gives it the ability to be accurate in its predictions and to stand behind that accuracy. Employers will receive regular data visualizations and guided data deep dives that demonstrate the measurable impact. They will observe the outcomes at work and among their workforce. They can finally move past the PhD theoretical models of validity and experience true efficacy.